41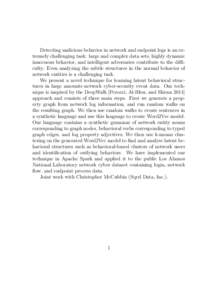 | Add to Reading ListSource URL: mmds-data.orgLanguage: English - Date: 2016-06-23 15:50:48
|
---|
42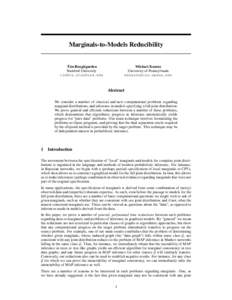 | Add to Reading ListSource URL: theory.stanford.eduLanguage: English - Date: 2013-11-08 17:46:03
|
---|
43![epl draft Scale-free networks as an epiphenomenon of memory F. Caravelli1 , A. Hamma2 and M. Di Ventra3 arXiv:1312.2289v5 [physics.soc-ph] 15 Feb 2015 epl draft Scale-free networks as an epiphenomenon of memory F. Caravelli1 , A. Hamma2 and M. Di Ventra3 arXiv:1312.2289v5 [physics.soc-ph] 15 Feb 2015](https://www.pdfsearch.io/img/39512c0565a6877f1afcfc739f5c2edc.jpg) | Add to Reading ListSource URL: arxiv.orgLanguage: English - Date: 2015-02-16 21:04:27
|
---|
44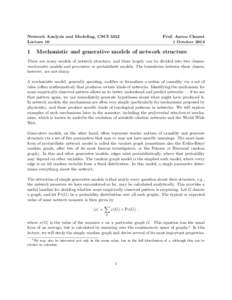 | Add to Reading ListSource URL: tuvalu.santafe.eduLanguage: English - Date: 2013-11-23 11:09:17
|
---|
45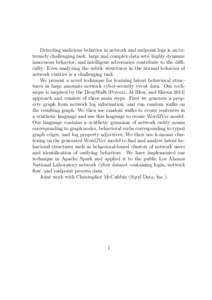 | Add to Reading ListSource URL: mmds-data.orgLanguage: English - Date: 2016-06-23 15:50:48
|
---|
46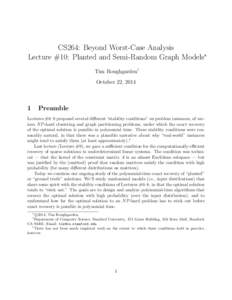 | Add to Reading ListSource URL: theory.stanford.eduLanguage: English - Date: 2015-01-05 12:59:35
|
---|
47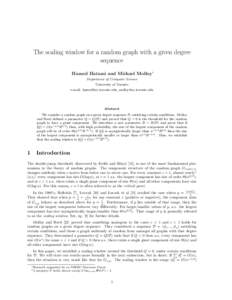 | Add to Reading ListSource URL: www.cs.toronto.eduLanguage: English - Date: 2011-11-23 23:07:53
|
---|
48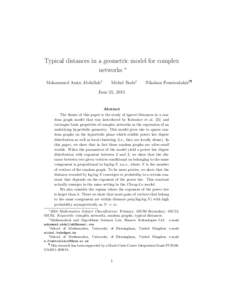 | Add to Reading ListSource URL: web.mat.bham.ac.ukLanguage: English - Date: 2015-06-25 12:56:44
|
---|
49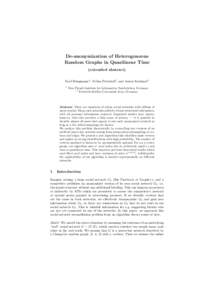 | Add to Reading ListSource URL: people.mpi-inf.mpg.deLanguage: English - Date: 2016-01-03 06:46:27
|
---|
50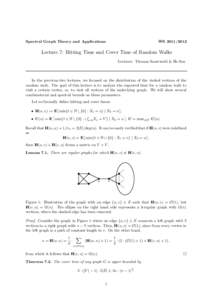 | Add to Reading ListSource URL: resources.mpi-inf.mpg.deLanguage: English - Date: 2011-12-08 09:45:37
|
---|